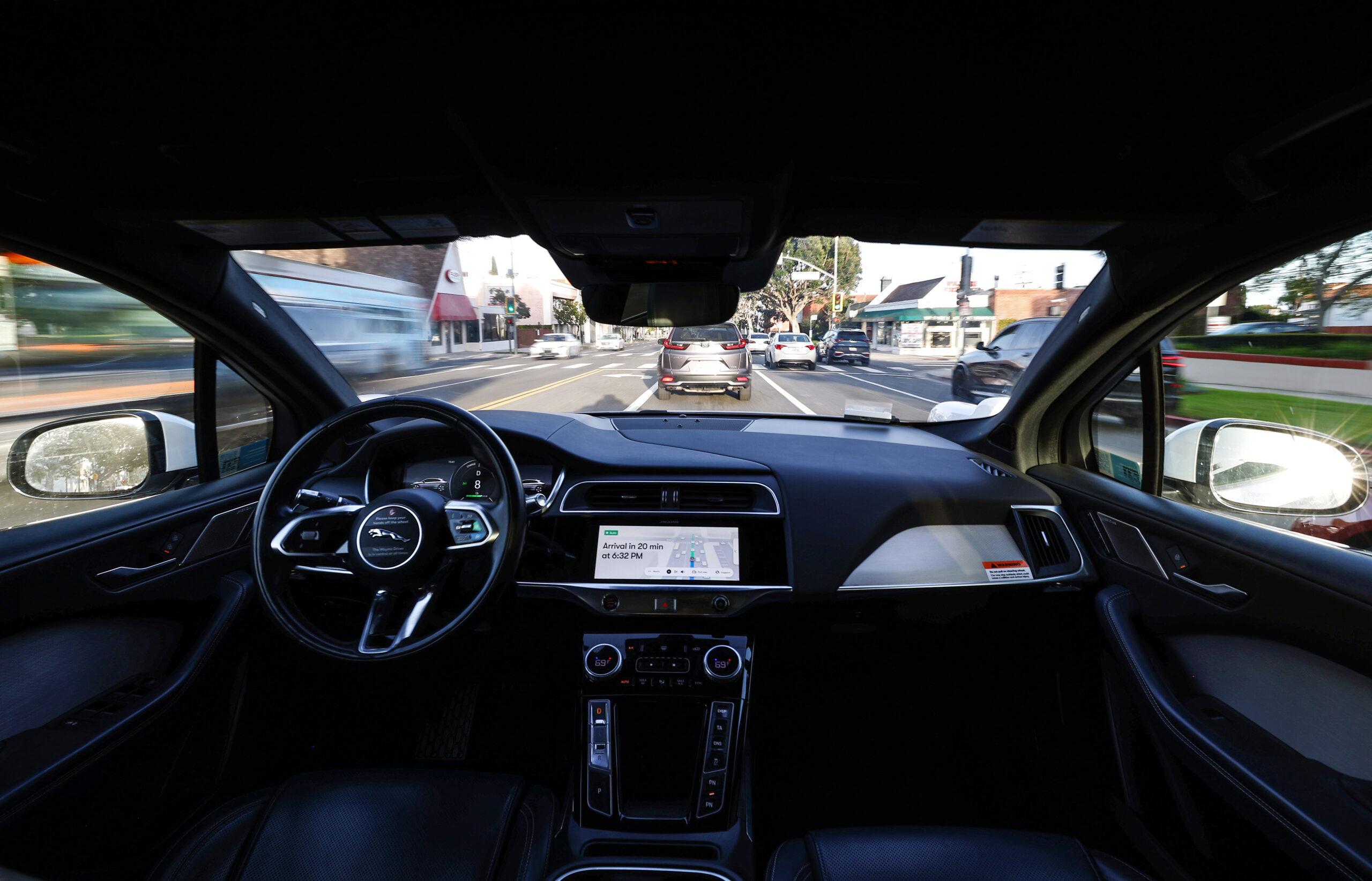
What would a world of self-driven cars look like? How would it change shopping, transportation, and life, more broadly?
A decade ago, many people were asking these questions, as it looked like a boom in autonomous vehicles was imminent. But in the last few years, other technologies—crypto, the metaverse, AI—have stolen the spotlight.
Meanwhile, self-driving cars have quietly become a huge deal in the U.S. Waymo One, a commercial ride-hailing service that spun off from Google, has been rolled out in San Francisco, Phoenix, Los Angeles, and Austin. Every week, Waymo makes 150,000 autonomous rides. Tesla is also competing to build a robo-taxi service and to develop self-driving capabilities.
There are two reasons why I’ve always been fascinated by self-driving cars: The first is safety. There are roughly 40,000 vehicular deaths in America every year and 6 million accidents. It’s appropriate to be concerned about the safety of computer-driven vehicles. But what about the safety of human-driven vehicles? A technology with the potential to save thousands of lives and prevent millions of accidents is a huge deal.
Second, the automobile was arguably the most important technology of the 20th century. The invention of the internal combustion engine transformed agriculture, personal transportation, and supply chains. It made the suburbs possible. It changed the spatial geometry of the city. It expanded demand for fossil fuels and created some of the most valuable companies in the world. The reinvention of last century’s most important technology is a massive, massive story. And the truth is, I’m not sure that today’s news media—a category in which I include myself—has done an adequate job representing just how game-changing self-driving technology at scale could be.
Today’s guest is Timothy Lee, author of the Substack publication Understanding AI. Today I asked him to help me understand self-driving cars—their technology, their industry, their possibility, and their implications.
If you have questions, observations, or ideas for future episodes, email us at PlainEnglish@Spotify.com.
In the following excerpt, Derek talks with Timothy Lee about the technology behind self-driving cars and how that compares to the technology that powers large language models such as ChatGPT.
Derek Thompson: I’d love you to make me smarter first about how self-driving cars work. I remember from my first ride in a Waymo maybe six or seven years ago out in the Bay Area, they’ve got a bunch of cameras mounted around this vehicle. It’s a normal car with a bunch of cameras on the top and on the sides, including the famous LiDAR machine, which creates a 3D image of the road.
Maybe it’s best to ask the question this way: How do self-driving cars perceive the world, and how do they use that perception to orient themselves through the world?
Timothy Lee: Sure. So, on the first question, how do they perceive the world, they’ve got a bunch of sensors, but the three most important are [cameras, radar, and LiDAR]. Cameras, which you mentioned, are just digital cameras just like you have on your phone. Radar, which is the same, is in some conventional cars and on boats and stuff; those are most useful for telling velocities. So, if you’re on the freeway and you wanted to see if the car ahead of you is decelerating, radars are very useful for that. And the third one, which is most unique to self-driving cars, is the LiDAR. The Waymo cars, for example, have these big spinning things at the top that have lasers. They fire laser beams in every direction several times a second, and they create a 3D point cloud.
And so this gives them a very precise map of all the physical objects in the world. And then they’ll combine the data from the LiDAR with the data from the cameras because the cameras will kind of give a visual of: What is this? And so they know there’s an object there, the camera helps them figure out what’s there, and then they end up with this 3D model of how the world works.
And so the software that does that first step of building the model, that’s usually called the perception part of the self-driving software, and then it does a lot of kind of internal simulation where it maps out: Here are some possible ways the future could unfold. And then, based on those possible scenarios, it figures out what’s the safest thing to do in the next second, the next two seconds, the next three seconds. It plans out a trajectory, and this ends up working pretty well.
Thompson: And how similar is the technology being used to make sense of the world? How similar is that to the transformer technology that powers large language models like ChatGPT?
Lee: So this has been something that’s been changing in the industry recently. I think we’ll talk later about how this industry has evolved. But self-driving cars have been around for about 15 years. The transformers are only about six or seven years old. And so early on, they had different techniques, but in the last three or four years, the leading companies have all started using the transformer in their self-driving software, and the architecture is pretty similar. It’s the same basic approach. I don’t think we need to get into details of how it works.
But the transformer is very good at taking large amounts of data and extracting patterns from it. And what that has meant is that the more recent generations of self-driving car software do much more learning from experience. If you gather lots and lots of data of real-world human driving or real-world self-driving car driving with feedback on whether it did it right or not, it can then extract patterns from that and say, “OK, in this particular situation, I should be this far from the curb. I should give the driver ahead of me this much space.”
Because part of what’s difficult about driving is there are the rules you see in the handbook, but then there are also lots of kind of subtle rules of thumb and dynamic kind of situations that are really hard to write explicit software rules. And so neural networks, in particular transformers, are really good at just looking at how have people done this in the past and figuring out implicitly what’s the rule that the human is following here and then doing a similar action that makes the car drive as if it seems like it’s a human driver.
Thompson: Yeah. It’s kind of funny to think about an analogy between ChatGPT getting smart about the world by reading Reddit, by reading human impressions of what is true and untrue, and a car making sense of the world. Rather than reading Reddit, it’s reading the road. It’s reading human interactions. It’s reading the fact that sometimes that pedestrian looking the other way will sometimes walk into the street. And so you have to slow down as you’re reaching the intersection because pedestrians don’t have perfect LiDAR awareness of what’s around them and they’ll walk in the middle of the street. And so they’re essentially reading the world and processing the world in a way that is similar to, in some ways, the way that ChatGPT makes sense of the corpus of the internet.
Lee: Yeah. One of the places the transformers have been most useful is: The earlier generations of self-driving cars really had trouble at intersections with a lot of different vehicles because to know what you should do, you have to predict what the other cars are going to do. But then what they are going to do is going to depend on what you do and what the other cars do. And so you have this computational explosion of the number of possible permutations you have to think of. And it’s a little bit like a conversation the cars are having, where each car sort of makes a move and then the other ones respond.
And so, in the same way that an LLM predicts the next word, what the transformer does in the prediction part of this software is it kind of predicts the next move. It says, “OK, each car gets a move,” and we build a network that kind of learns the strategy that each car plays, and then it kind of plays this game out inside a data center inside a computer. And they found that that worked much better than a computer programmer trying to explicitly write out, “OK, the rule each car should follow is x,” because it’s very complicated, and you have this kind of computational explosion if you try to do it very explicitly.
This excerpt was edited for clarity. Listen to the rest of the episode here and follow the Plain English feed on Spotify.
Host: Derek Thompson
Guest: Timothy Lee
Producer: Devon Baroldi
Subscribe: Spotify